Artificial General Intelligence (AGI) has long been a theoretical concept, evoking both excitement and dread. Recent developments suggest it may not remain theoretical for much longer.
OpenAI CEO Sam Altman has declared on his personal blog that his team “knows how to build AGI as we have traditionally understood it.” Altman’s confidence is evident in OpenAI’s pivot toward an even grander ambition: Superintelligence. As he notes in the blog post, “We are beginning to turn our aim beyond [AGI], to superintelligence in the true sense of the word.”
This marks a turning point in how we think about artificial intelligence – and for many, it’s as thrilling as it is terrifying.
For the life sciences industry, AGI and its successor, Artificial Superintelligence (ASI), could herald nothing less than a revolution. But these developments come with a cascade of ethical, logistical and existential questions. What happens when machines think faster, better and more broadly than any human ever could? And what can life sciences organizations do to prepare for the inevitable?
Pharma is largely bullish on these technologies and a great majority of what comes with them. “AGI has the potential to revolutionize commercial strategy in pharma by helping connect real-world global health datasets to solve complex health challenges. This can help tailor treatments to similar patient populations more effectively, but new regulation frameworks and guidelines will be required in this space,” says Niveditha Mogali, Director, Enterprise Omnichannel Analytics Capabilities, at GSK. “It can also transform care through personalized AI assistants for patients by providing education, resources and health monitoring based on individual patient needs.”
Transformative shifts
In the years leading up to Altman’s statement, the field of artificial intelligence had already undergone several transformative shifts. From the early days of rule-based “expert systems,” researchers made significant strides in machine learning and eventually deep learning – techniques that allowed computers to learn patterns from massive datasets, rather than relying on explicitly programmed instructions. The breakthrough success of neural networks in image recognition, natural language processing and game-playing (remember AlphaGo’s historic victory over world champion Go player Lee Sedol?) showed that AI had moved from a niche research topic to a technology reshaping entire industries.
During this period, the rise of large language models (such as OpenAI’s GPT series) and image generators (including DALL·E and Stable Diffusion) grabbed public attention and fueled commercial interest. These models demonstrated a remarkable ability to generate and understand language or create sophisticated images from text prompts, the former a feat once deemed impossible outside of tightly controlled lab environments. Yet despite these leaps, the AI systems were still largely considered narrow; each excelled at a specific task but lacked the broader adaptability of human intelligence.
This progress set the stage for more ambitious goals. Researchers began to talk not just about solving specific problems but also about building AI that could learn, reason, and perform across myriad domains—a concept known as Artificial General Intelligence (AGI). However, for many experts, AGI remained a distant milestone, something to be speculated on rather than actively pursued. That is, until Sam Altman’s statement brought the conversation out of the realm of “maybe someday” and into the here and now, prompting an industry-wide reassessment of both the promise and peril that AGI might bring.
Breaking down AGI and ASI
AGI refers to an AI system that can perform any intellectual task a human can, across various domains, without needing to be retrained. Today’s AI systems are excellent at specialized tasks – for example, analyzing medical images or predicting drug interactions – but AGI can adapt and apply knowledge across disciplines much like a human scientist.
ASI takes this concept even further. It refers to AI that surpasses human intelligence and operates at a level we can barely comprehend. While AGI might collaborate with researchers to enhance human efforts, ASI could independently lead scientific revolutions, reshaping entire fields of study in the process.
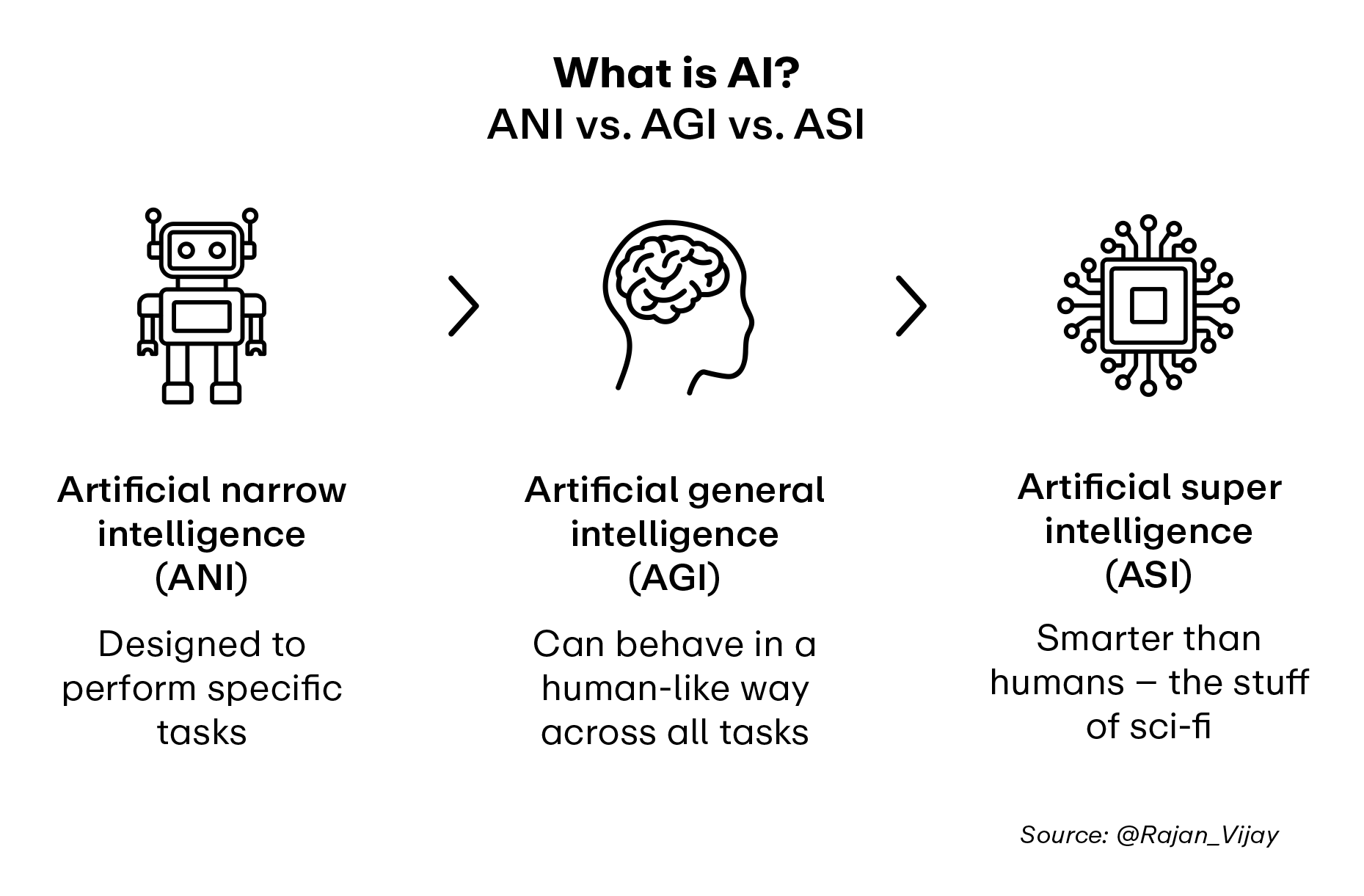
Accelerated discovery in biopharma
AGI’s most immediate and transformative effects in life sciences will likely be in drug discovery and development. Traditional pharmaceutical R&D takes over a decade and costs billions of dollars. AGI could slash these timelines and costs by analyzing vast datasets, generating new hypotheses and designing novel compounds – all without human intervention.
Current AI systems already assist in identifying potential drug candidates, but AGI could autonomously predict how compounds interact across complex biological systems. Imagine treatments for rare cancers or Alzheimer’s disease emerging not over years but months, thanks to AGI’s unparalleled computational abilities.
Rethinking clinical trials
Clinical trials, often hampered by logistical challenges and recruitment hurdles, could similarly undergo a seismic shift. By modeling human biology with extreme accuracy, AGI could simulate clinical trials virtually, reducing the need for extensive (and costly) studies.
This could significantly accelerate the timeline for bringing new therapies to market. AGI could also identify niche patient populations for rare diseases, ensuring trials are more targeted and effective. This, in theory, would benefit both patients and pharmaceutical companies.
Personalized medicine at scale
AGI’s capacity to synthesize information from genomics, patient records and clinical data could make true personalized medicine a reality. By rapidly identifying genetic markers, lifestyle factors and environmental influences, AGI could design tailored treatments for every patient with unprecedented precision.
Picture a future in which AGI crafts individualized cancer treatment plans, optimizing drug combinations and dosing schedules in real time. This is no longer a distant dream; it’s a possibility within the next decade.
Ethical and regulatory implications
While AGI’s potential is vast, it raises profound ethical and regulatory questions. How do we govern systems that can outthink and outperform human researchers? How can we ensure that the data these systems use is unbiased and inclusive?
Life sciences organizations will need to collaborate with regulatory bodies to develop frameworks that keep pace with these advancements. Transparency, interpretability, and ethical AI development must become foundational to the adoption of AGI. Without such safeguards, the industry risks both public backlash and the unintended consequences of opaque AI systems.
Spotting the winners
Trends on prediction markets such as Kalshi underscore that AGI isn’t just a theoretical possibility; it’s coming, and soon. But how can industry leaders identify the companies most likely to capitalize on these advancements? Look for organizations investing heavily in AI-driven R&D, those forging partnerships with AI pioneers and those embracing business models that integrate these technologies.
Companies in the realms of rare disease, oncology and regenerative medicine are especially well-positioned to lead, given the complexities and unmet needs in these areas. Those breaking away from the pack may already be laying the groundwork for AGI’s arrival.
***
So where will AGI’s impact be felt first? Likely in areas like drug discovery, personalized medicine and diagnostics, fields where complex datasets and systemic inefficiencies are ripe for disruption.
For life sciences leaders, the question isn’t if AGI will arrive, but when. The future may feel uncertain, but one thing is clear: Those who embrace AGI and ASI today will shape the next chapter of human health.
Has your organization started to pursue opportunities around AGI and ASI? Drop us a note at [email protected], join the conversation on X (@KinaraBio) and subscribe on the website to receive Kinara content.