We are living through the unfolding test of a bold hypothesis: That scaling compute and model size alone is enough to reach general-purpose intelligence.
So far, the hypothesis hasn’t been broken. Bigger models keep getting better. GPT-2 was a toy. GPT-4 can write Python scripts, reason through clinical vignettes and summarize research papers. And if the scaling laws hold for another few orders of magnitude, we may soon reach models that can do meaningful scientific research and development—possibly better than humans.
If that happens, the implications for life sciences aren’t just big—they’re structurally destabilizing. AI won’t just support pharma R&D; it could become the primary driver of it. But how fast this happens, and how unevenly it plays out, remains deeply uncertain.
This piece lays out two plausible trajectories—an explosive takeoff versus a slower climb—and what each would mean for drug discovery, trial design, marketing and regulatory engagement. The core premise is what the former OpenAI employee Leopold Aschenbrenner terms “situational awareness”: If you’re a decision-maker in life sciences, you don’t need to bet on one future. You need to be ready for both.
Scaling Is Working, and the Curve Hasn’t Bent Yet
The most surprising thing about the evolution of AI during the last five years is how unsurprising it’s been, at least to those paying attention to scaling curves. As model size and compute increase, capabilities have improved in mostly smooth, continuous ways. There have been no magical breakthroughs and no radical new algorithms, just brute-force scaling of transformers across massive data sets and GPU clusters.
GPT-2, released in 2019, was incoherent but interesting. GPT-3, released in 2020, was surprisingly articulate. GPT-4, which debuted in 2023, remains useful across a wide range of cognitive tasks. When you plot performance on a log-log chart against compute, the line has remained stubbornly straight.
This doesn’t guarantee that the next jump will be as fruitful as the last. There may be limits in the form of data bottlenecks and energy constraints. But so far, the empirical trend is holding. If that continues, by 2027-2028 it’s plausible we’ll see AI models capable of autonomous scientific reasoning. It’s not general intelligence in the mythic sense, but something close enough to reshape entire industries.
Two Futures: Fast Takeoff and Gradual Ramp-up
Forecasting AI timelines is notoriously difficult. But for life sciences strategy, binary framing is still useful: What happens if the AI curve bends hard upward? versus What happens if it stays on its current slope?
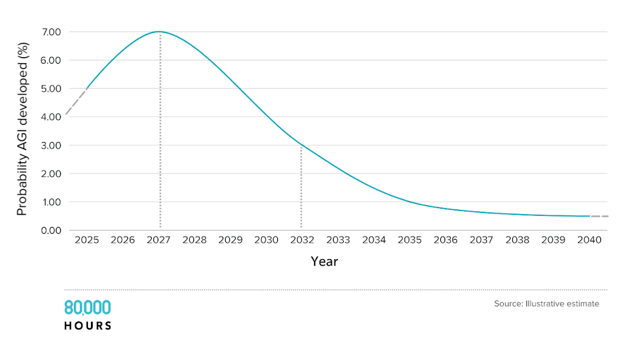
(80,000 Hours’ illustrative estimate of the probability that artificial general intelligence is developed over the next two decades)
In a fast-takeoff scenario—considered by some to have a 20-30% likelihood—the scaling laws continue unimpeded. Models trained in 2027 aren’t just better than GPT-4; they’re qualitatively different. These systems can design, test, and optimize molecular structures in simulation, run high-fidelity virtual clinical trials and replace large swaths of human cognition in R&D and analytics. As soon as AI can do AI research, recursive self-improvement becomes possible. This may not be world-ending, but it could compress decades of biotech innovation into just a few years.
Life sciences organizations in this world would face a brutally fast reshuffling. Old pipelines would become obsolete. The marginal cost of a “digital scientist” would drop to pennies. Teams without AI-native infrastructure and fluency would be left behind. This future feels like a regime change: Once it starts, it’s already too late to adapt.
In a slower-takeoff scenario—which experts peg at a 70-80% probability—AI continues improving rapidly but non-catastrophically. We see yearly gains in model capability, steady integration into workflows and lots of experimentation, some of which results in dead ends. AGI might arrive in the 2030s rather than the 2020s. In the meantime, we get increasingly useful narrow systems: Co-pilots for drug discovery, auto-generated trial protocols and personalized medicine interfaces.
This slower world is less disruptive and arguably more stable. Organizations can incrementally upskill teams, build clean data systems and form long-term partnerships with AI vendors. It’s still a wild ride—but it’s a hill, not a cliff.
What Changes Either Way: R&D, Trials and Personalization
Regardless of speed, the direction is clear: AI is reshaping life sciences from the inside out.
R&D is becoming machine-augmented. Early-stage drug discovery is already benefiting from deep learning in areas like protein folding (via AlphaFold), molecule generation (through tools like MolDx), and literature mining using NLP. Next-generation models could autonomously generate hypotheses from the full corpus of biochemical literature, design molecules with better on-target and off-target profiles, and simulate in vitro results before any wet lab experiments. We’re not there yet—but once we are, drug design may shift from “hit or miss” to “query and optimize.”
Clinical trials, too, become data problems. Recruitment, inclusion criteria and dropout modeling are fundamentally pattern recognition tasks. As such, they are well-suited to AI. These systems could predict responders using EHRs and genomics, simulate outcomes under different protocols and flag early signs of adverse events. Eventually, we may achieve in silico trials validated against real-world outcomes. This isn’t science fiction; it’s an engineering and regulatory challenge.
Personalized medicine, long more of a marketing slogan, may become the default. The vision is an AI system that integrates genomics, labs, wearables and social determinants to recommend the right treatment for the right patient at the right time. At scale, this will require robust multi-modal data, patient-level modeling and clinical validation pathways. But the payoffs—better outcomes, lower costs, less trial-and-error—are difficult to overstate.
Marketing and Communication in the Age of Intelligence
Marketing will not be immune to this transformation. In fact, it may be one of the earliest domains to be fundamentally rewired by AI. Models will serve as both message generators and subjects of the message itself. This means personalized content and adaptive campaigns fine-tuned for each audience segment. It also means that being able to explain what your AI-enabled product actually does becomes a strategic competency.
This will create a literacy divide: Some stakeholders will grasp what your model does, while others won’t. You’ll need to bridge that gap, not widen it. Companies will thus want to avoid the twin failure modes of overhyping (“our AI finds cures”) and underselling (“it’s just a tool”). Instead, they should focus on validated outcomes, real-world value and clear explainability.
Assume your audience is growing more skeptical and more technically literate. Earn their trust with evidence.
Regulatory and Ethical Time Horizons
No amount of scaling can bypass governance. The key question is not whether regulators will respond, but how fast and how wisely.
Upcoming pain points include how to validate an AI-generated molecule, how to assign liability if an AI suggests an incorrect protocol and what qualifies as “explainable” in a large language model’s recommendation. The best-case scenario is that the industry and regulators co-create standards before chaos hits. That means early engagement, shared data and audit tools—and internal ethics boards.
Failing that, we may face blunt moratoriums and wasted potential. Here’s an idea: Treat explainability as a core product feature, rather than a compliance chore.
What to Do Now: Decision Hygiene for Uncertain Futures
You don’t need to know which future will arrive, but you need to act in ways that are robust across both timelines. The best strategic moves that can be made immediately include cleaning your data (if your data is messy and siloed, you are far from alone), investing in AI fluency (not everyone needs to code, but everyone must reason clearly about AI), forming fast feedback loops through pilots rather than white papers, partnering with fast-moving labs and startups, and planning for both the three-year and 10-year AI timelines.
It’s tempting to forecast based on gut instinct or industry mood, but the smarter move may be to treat the future like a Bayesian update loop. AI is scaling fast. While general capability is not guaranteed, it’s more probable than many institutions are anticipating.
If you’re in life sciences, that gap—between the expected level of disruption and current preparedness—is where most of the risk, and most of the opportunity, lies.
The models are learning. The question is: Are you?
Is your organization prepared for both the explosive and steady growth trajectories for general-purpose intelligence? Drop us a note at [email protected], join the conversation on X (@KinaraBio) and subscribe on the website to receive Kinara content.
***
Sources and additional reading:
Situational Awareness: The Decade Ahead
AI 2027
The Scaling Era: An Oral History of AI, 2019-2025
Preparing for the Intelligence Explosion
The Case for AGI by 2030
Digital People FAQ